Use Case Financial
Propensity Models and Creation of Sustainable Data Repositories
The main objective of the sustainability project is to implement an advanced data-driven analysis system that enables the banking entity to optimize energy efficiency and promote sustainable mobility within its customer base. This is achieved through the collection and processing of structured data in tables, which generate predictive models aimed at identifying behaviors related to energy consumption, the adoption of sustainable technologies (such as solar panel installation or the purchase of electric vehicles), and the evaluation of environmental impact.
The developed models provide valuable insights for designing sustainable financial products, as well as for personalizing marketing campaigns and business strategies aimed at promoting more eco-friendly practices among clients. Additionally, the system is flexible and scalable, allowing for the incorporation of new sustainability attributes without the need to restructure the existing database, thus facilitating strategic decision-making in the future.
Challenges
Successfully implementing sustainability initiatives requires addressing challenges such as ensuring data quality, standardizing global processes, and integrating technical and functional requirements. These solutions help create accurate, scalable models that provide consistent insights and support effective decision-making across regions.
Data quality and completeness
Ensuring data integrity in propensity models is critical. Variability in data quality and completeness across different geographies can affect the accuracy of analyses and predictions.
Effective global processes
Defining and applying global processes that adapt to local dynamics and are replicable in different regions without losing accuracy.
Technical and functional integration
Coordinating technical requirements with the functional needs of each model is essential to accurately reflect the operational reality in different regions.
Sustainability data repository
Creating a centralized repository to manage and standardize key sustainability data, facilitating uniform access and use across geographies.
Solution
Propensity Models: Definition of models for sustainable products (solar panels, eco-friendly cars, generic cars, and vehicle financing) to predict and optimize customer behavior aligned with the bank’s sustainability goals.
Data Repository: Implementation of a centralized repository that standardizes and manages sustainability attributes, improving data quality and consistency across geographies.
Extrapolable Dashboards: Development of dashboards that allow data integration into the Data Management System (SDM), facilitating the globalization and replicability of the models.
Technical and Functional Validation: Assessment of the integrity of data solutions between countries to ensure technical and functional consistency, aligning the models with global sustainability goals.
Documentation and Processes: Creation of detailed documentation and definition of processes for the autonomous implementation and adaptation of models in different geographies.
Tech stack
Results
The sustainability project has enhanced data consistency, streamlined global implementation, and optimized propensity models for sustainable products like solar panels and eco-friendly cars. These improvements have led to more accurate predictions, faster deployment, and quicker issue resolution, boosting overall project efficiency and decision-making.
Afterward, an SVM model was applied to further enhance the results.
Data consistency
The sustainability data repository has provided a homogeneous and reliable source, significantly reducing quality and completeness errors in propensity models.
Global implementation efficiency
The standardization of processes and the creation of extrapolable dashboards have streamlined the implementation of sustainability models, shortening the deployment time.
Optimization of propensity models
The models for solar panels, eco-friendly cars, and vehicle financing have increased the accuracy of predictions, enhancing the bank’s decision-making in sustainable products.
Reduced issue resolution time
Technical validation and rapid resolution of functional issues have reduced the response time to incidents, increasing project efficiency.
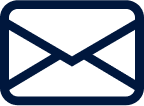