Use Case Oil & Gaz
Predictive Maintenance
Refineries use complex processes involving multiple industrial machines and equipment. The malfunction or failure of this equipment has a direct impact on production.
Our client wants to model the degradation processes of its equipment to avoid breakdowns.
Challenges
Implementing predictive maintenance in refineries requires addressing key challenges such as accurately modeling equipment degradation, minimizing unexpected downtime, and effectively leveraging sensor data for predictive insights.
Degradation Modeling
Develop a system capable of modeling the degradation processes of hydrocracker equipment
Minimizing Downtime
Minimize unplanned downtime due to anomalies or failures
Data-Driven Insights for Predictive Maintenance
Implement an efficient data collection and analysis framework to extract meaningful insights from sensor data, improving failure prediction accuracy.
Solution
Development of predictive models and advanced analytics, integrated with IoT & Edge.
Collect data generated by more than 300 sensors for one year.
Statistical analysis to identify key variables (seal pressure, equilibrium line, suction pressure, etc.) impacting pump degradation based on linear and nonlinear correlations.
Evaluation of multiple predictive techniques (statistical, supervised or unsupervised models, neural networks) to identify the most appropriate model.
Integration of models and results into reporting tools with automated notifications.
Results
The final model is based on the 2 Level Rolling Deviation statistical methodology and was developed in Python and on the AWS cloud platform.
54
Identified predictive variables to characterize the degradation process
1.5M$
Estimated savingsby identifying and preventing unplanned downtime
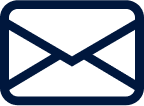