Use Case Financial
Interdependencies & conditional probabilities
The “Causal ML” mission at a leading player in the financial sector aims to analyze the interdependencies between risks databases to establish causal chains and clusters. The goal is to improve risk consolidation and inform the organization’s internal capital calculation.
Challenges
Understanding risks database
Understand the risk database to select key variables and gain an overall view of the causal relationships between events.
Estimating probabilities without historical data
Estimate prior and conditional probabilities despite the lack of historical data, with only the probabilities of events available.
NLP library challenges
The lack of access to certain necessary NLP libraries to capture the complex relationships between events and their triggers limited the analysis and modeling.
Adapting to AI constraints
Adapt to our client AI constraints during industrialization by adjusting models to meet technical and security requirements while optimizing the integration of solutions into existing systems.
Solution
Analysis of the risk database: We studied the structure of the database and used Bayesian networks to model the interdependencies, specifically selecting “events” and “triggers” as key components of the Bayesian network.
Probability estimation: In the absence of historical data, we estimated the probabilities of “triggers” by using the frequency and imminence of “events” related to them. We then applied a numerical optimization algorithm to estimate conditional probabilities.
Fuzzy matching algorithm: We employed a fuzzy matching algorithm to achieve satisfactory results, with the potential to integrate other NLP approaches in the future.
Adaptation to AI constraints: We adjusted the models to meet client’ technical and security requirements, ensuring smooth integration of solutions into existing systems.
Tech stack
Results
The project led to the identification of new risk drivers and the mapping of relationships between “events” and “triggers.” We also estimated the direct and indirect costs associated with these risk events. Additionally, clusters and causal chains were detected to better understand the interdependencies among the risk factors. Finally, risk prediction was enhanced by incorporating business knowledge through simulations using the Bayesian network.
+30%
Estimated costs linked to detected risks by accounting for causal relationships.
40
Clusters detected (based on the parameters defined in our simulation).
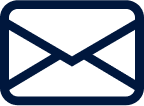